Introduction
Artificial Intelligence (AI) is a subfield of computer science that aims to create machines capable of mimicking human cognitive functions, such as learning, problem-solving, and decision-making. According to the Association for the Advancement of Artificial Intelligence, AI is defined as “the scientific understanding of the mechanisms underlying thought and intelligent behaviour and their embodiment in machines.” Essentially, AI technologies are designed to perform tasks that would normally require human intelligence. These tasks range from simple operations like recognizing patterns and sorting data to complex feats like diagnosing diseases, driving cars, or even contributing to scientific research. Understanding AI is increasingly important as it begins to play a significant role in various aspects of our daily lives.
The Ancient Allure of Artificial Beings
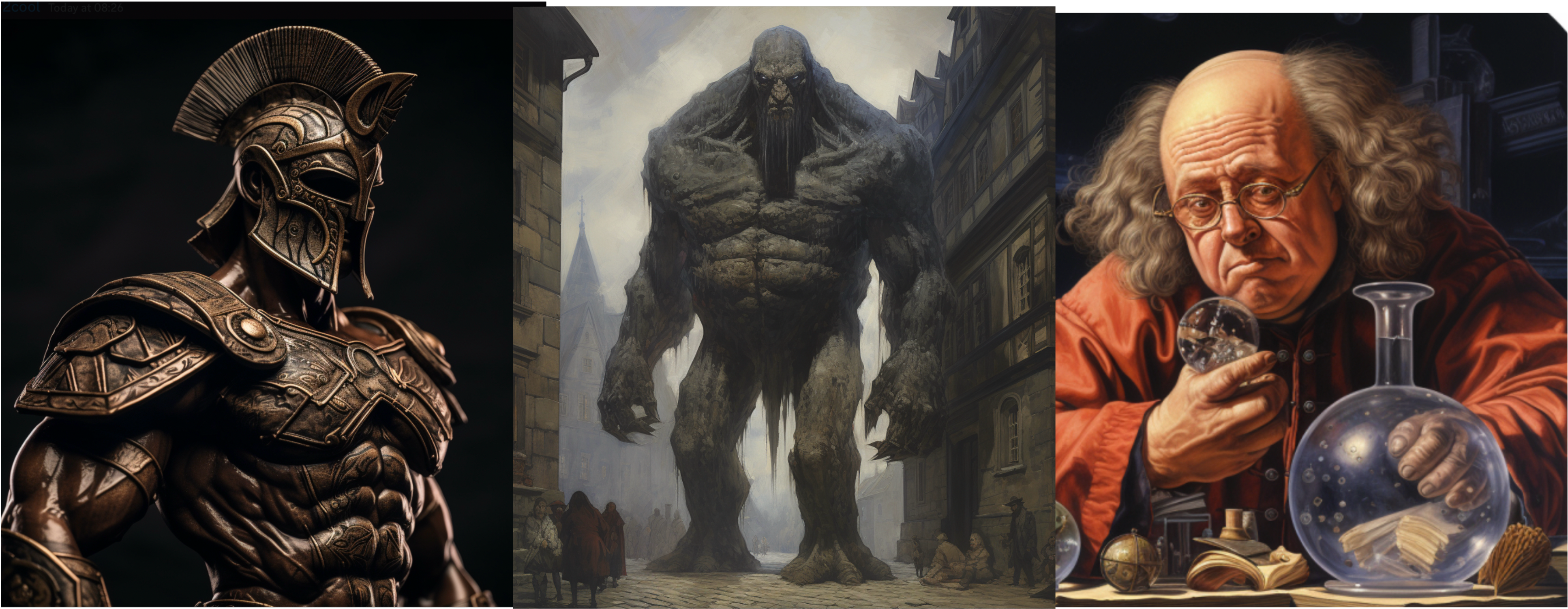
Long before the advent of modern Artificial Intelligence, humanity has been captivated by the idea of creating life-like beings through artificial means. Myths and legends from various cultures offer early glimpses into this fascination. For example, in ancient Greek mythology, Talos was a giant bronze automaton created by Hephaestus, the god of metallurgy, to protect the island of Crete. Jewish folklore speaks of the Golem, a clay figure animated through mystical means to serve and protect its creators. Similarly, the concept of the Homunculus, stemming from alchemical traditions, is an artificially created miniature human that embodies the human desire to create and understand life. These age-old stories reveal the long-standing human dream of mastering the creation of intelligent entities, a dream now being realized through the advances in Artificial Intelligence.
Early Milestones in Computational Thinking
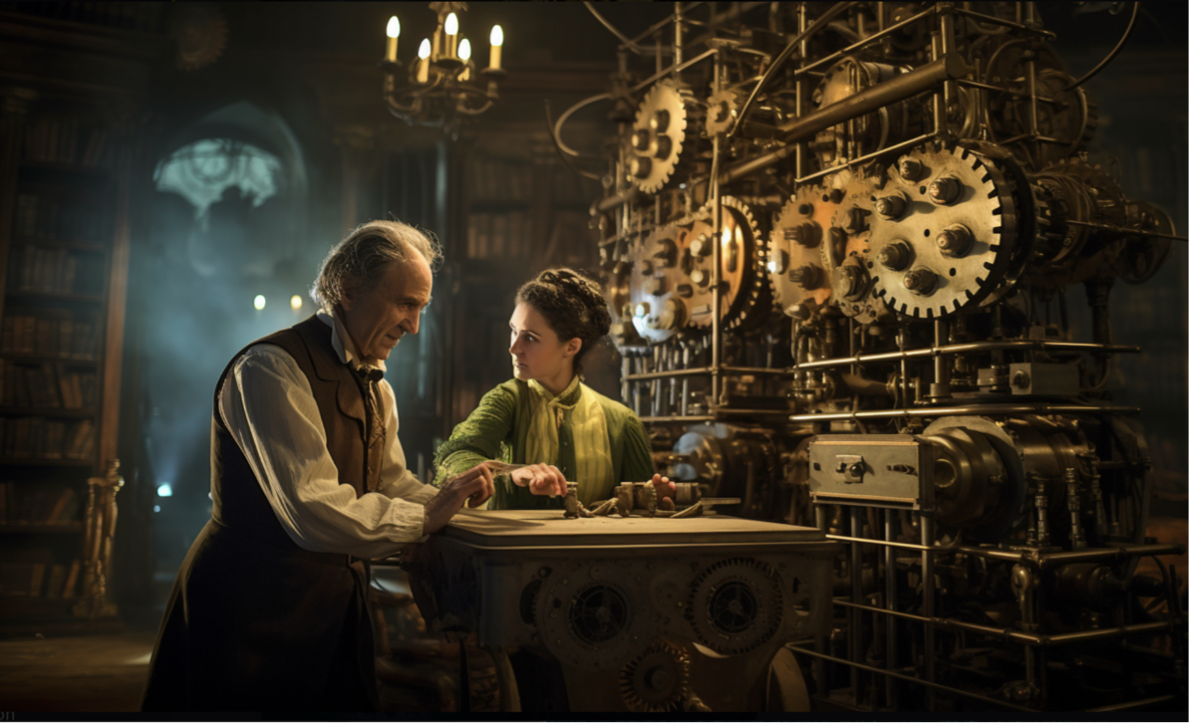
The journey toward developing machines that could aid or mimic human cognition has deep historical roots. One of the earliest known devices created for computation is the abacus, dating back to ancient civilizations. This simple tool helped humans perform mathematical calculations more efficiently. During the Victorian era, society was enchanted by ‘automata’—intricate mechanical devices that mimicked human or animal movements, often used for entertainment. The fascination with machine-aided thinking took a giant leap forward in the 19th century with Charles Babbage and Ada Lovelace. Babbage conceived the idea of the “Analytical Engine,” a machine designed to perform complex calculations. Lovelace, often considered the world’s first computer programmer, recognized that Babbage’s invention had the potential for not just arithmetic but also creating any content, including music or art. Their work laid the intellectual groundwork for the modern field of computer science and, by extension, Artificial Intelligence.
Isaac Asimov and the Laws of Robotics
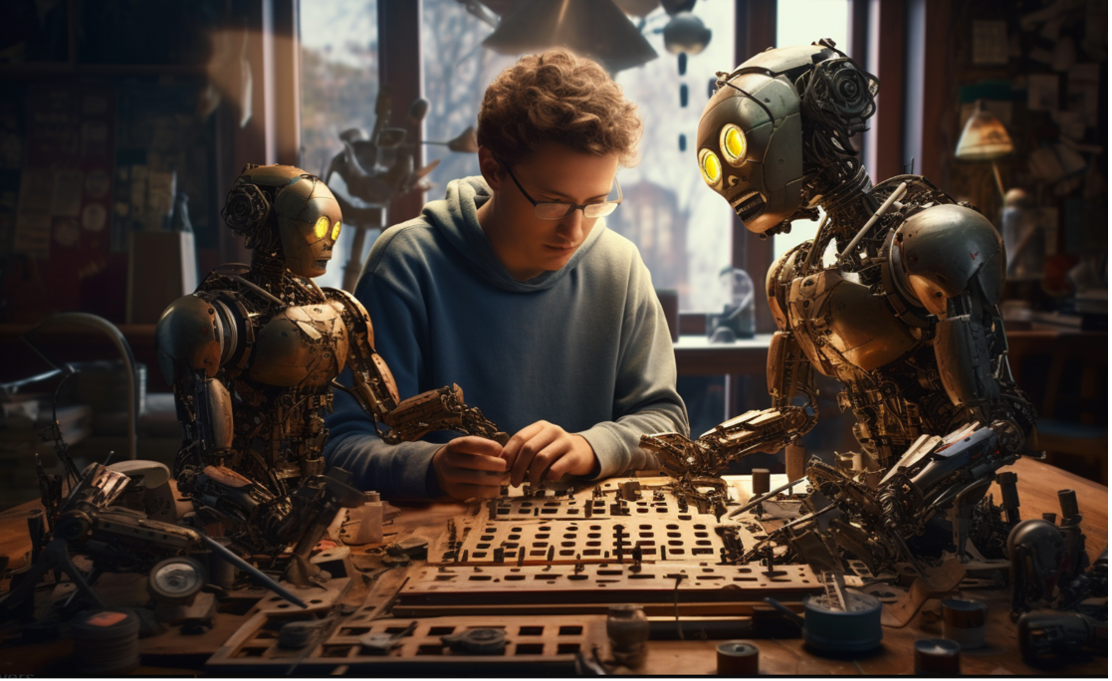
One of the seminal figures in conceptualizing the relationship between humans and intelligent machines is Isaac Asimov, a prolific science fiction writer and biochemist. In 1942, Asimov introduced the “Three Laws of Robotics” in his short story “Runaround,” part of the collection titled “I, Robot.” These laws were designed to govern the behaviour of artificially intelligent robots, with the primary aim of ensuring human safety. The laws are: 1) A robot may not injure a human being or, through inaction, allow a human being to come to harm; 2) A robot must obey the orders given it by human beings, except where such orders would conflict with the First Law; 3) A robot must protect its own existence as long as such protection does not conflict with the First or Second Law. Asimov later added a “Zeroth” Law that prioritizes humanity’s welfare above all. These laws have transcended fiction and are often cited in ethical discussions surrounding AI and robotics, illustrating the ways in which imaginative literature can shape real-world ethical and technical considerations.
Wave 1: Symbolic Problem Solving and the Dawn of AI
The first wave of Artificial Intelligence, often categorized as ‘symbolic AI,’ can be traced back to foundational thinkers like Alan Turing, who posed the question, “Can machines think?” in the 1950s. The invention of transistors provided the necessary hardware to convert logical reasoning into machine operations. Pioneering algorithms emerged, facilitating computational problem-solving. One pivotal moment in AI history was the Dartmouth Workshop in 1956, which is widely considered the birth of AI as a field of study. During this era, Arthur Samuel developed a machine capable of playing checkers, employing techniques that allowed it to learn from its experiences. Projects like the “Logic Theorist,” often considered the first AI program, and “Shakey the Robot,” one of the earliest examples of robotics, showcased the potential for machines to mimic human-like problem-solving. Early programming languages like Fortran and Lisp were crucial tools for codifying complex algorithms. This wave set the stage for AI, blending insights from computer science, logic, and even philosophy to establish the field’s initial framework.
The AI Winter of the 1970s: A Cautionary Interlude
After the promising advances of the first wave, the field of AI entered a period of stagnation and reduced funding known as the “AI winter” during the 1970s. The lofty expectations set by early successes were not immediately met, resulting in disillusionment both in academic circles and among potential investors. Several factors contributed to this setback: limitations in computing power, lack of sophisticated algorithms, and challenges in scaling up existing technologies. This period served as a humbling reminder that the pathway to fully realized AI was fraught with complexities and hurdles. Despite this, the AI winter also paved the way for critical introspection and more realistic goal-setting, which would prove invaluable for the resurgence and advances that followed.
Wave 2: Expert Systems, Machine Learning, and Deep Blue
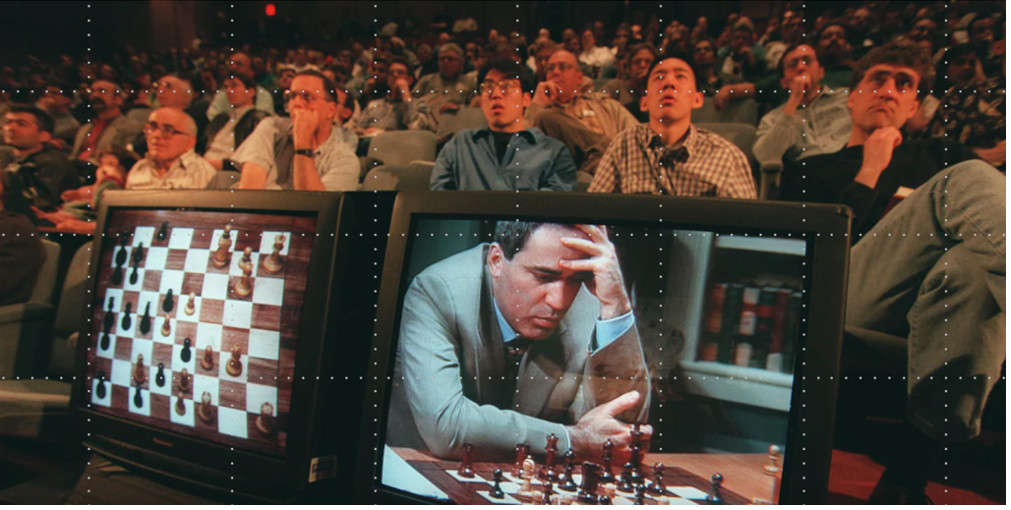
The second wave of Artificial Intelligence marked a shift from symbolic reasoning to specialized knowledge domains and machine learning techniques. During this phase, ‘Expert Systems’ emerged—computer programs designed to emulate the decision-making abilities of human experts in specific fields such as medicine or law. Alongside this, the Perceptron model, developed by Frank Rosenblatt, introduced a simplified neural network that could learn from data, laying the foundation for modern machine learning algorithms. Machine learning gained traction as computers became more powerful, enabling them to handle larger datasets and perform more complex calculations. Perhaps one of the most iconic milestones of this era was IBM’s Deep Blue defeating world chess champion Garry Kasparov in 1997. This event captured public imagination and signalled that machines could not only mimic human-like reasoning but also surpass human expertise in highly specialized tasks. The second wave was characterized by more pragmatic approaches and applications, and it set the stage for the immense possibilities that AI holds today.
Wave 3: Data-Driven AI and Technological Synergy
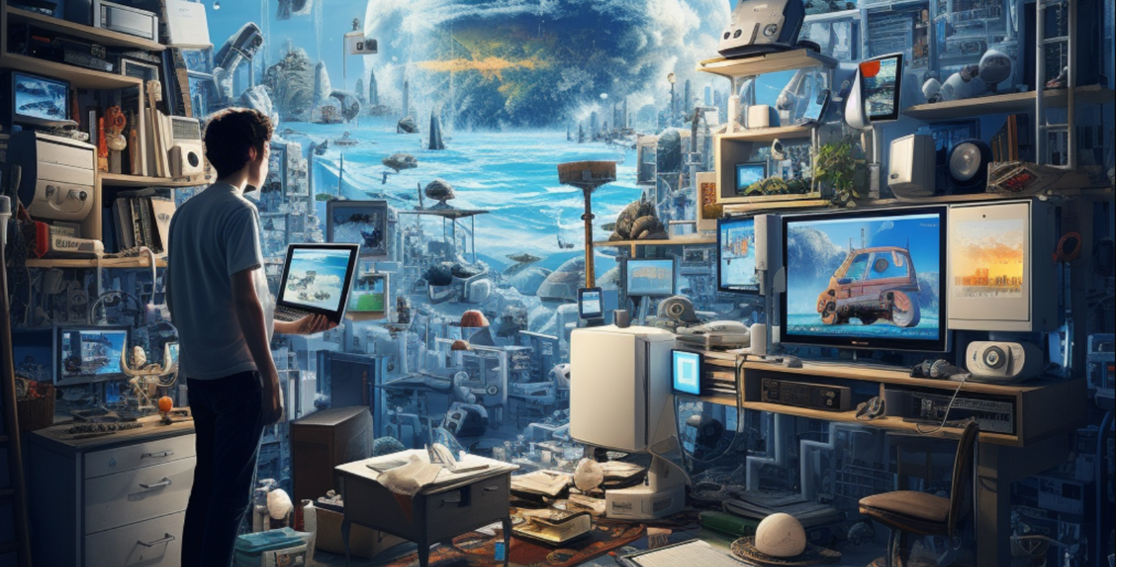
The third wave of Artificial Intelligence has been fuelled by an unprecedented explosion of data, facilitated by the internet, smartphones, and the Internet of Things (IoT). Coupled with this data boom are significant advancements in storage and computing capabilities—cloud computing, distributed file systems, and high-performance computing architectures like Graphics Processing Units (GPUs) have greatly accelerated the speed and scale at which AI can operate. This technological synergy has allowed for the practical application of sophisticated algorithms rooted in Bayesian statistics, a field inspired by Thomas Bayes, an 18th-century statistician and theologian. Deep learning, a subset of machine learning, has become the current state-of-the-art, enabling groundbreaking applications in natural language processing, computer vision, and automated decision-making. This third wave has not only expanded the boundaries of what AI can achieve but also ingrained it deeply into our everyday lives and critical systems.
Conclusion: The Double-Edged Sword of AI
As we stand on the cusp of an age increasingly dominated by Artificial Intelligence, it’s crucial to engage in a nuanced dialogue about its potential benefits and risks. On one hand, AI holds the promise of solving some of humanity’s most pressing challenges—medical diagnoses, climate modelling, and even contributing to social justice initiatives. However, these advancements come with their own set of ethical and practical concerns, including job displacement due to automation, data privacy issues, and the potential for AI systems to perpetuate societal biases. Moreover, the decision-making processes of complex AI algorithms can be difficult to understand, even for experts, leading to issues of transparency and accountability. As we navigate this exciting yet fraught landscape, it is imperative for society to approach AI with a balanced perspective, safeguarding against its risks while harnessing its incredible potential for good.
Terry Cooke-Davies
September 2023
Link to Web Page — Resources: Artificial Intelligence
This article was created with the assistance of ChatGPT Plus, and the original illustrations were created using Midjourney, an AI “text to graphics” programme.